7 4: Blocking in 2 Dimensions Latin Square Statistics LibreTexts
Table Of Content
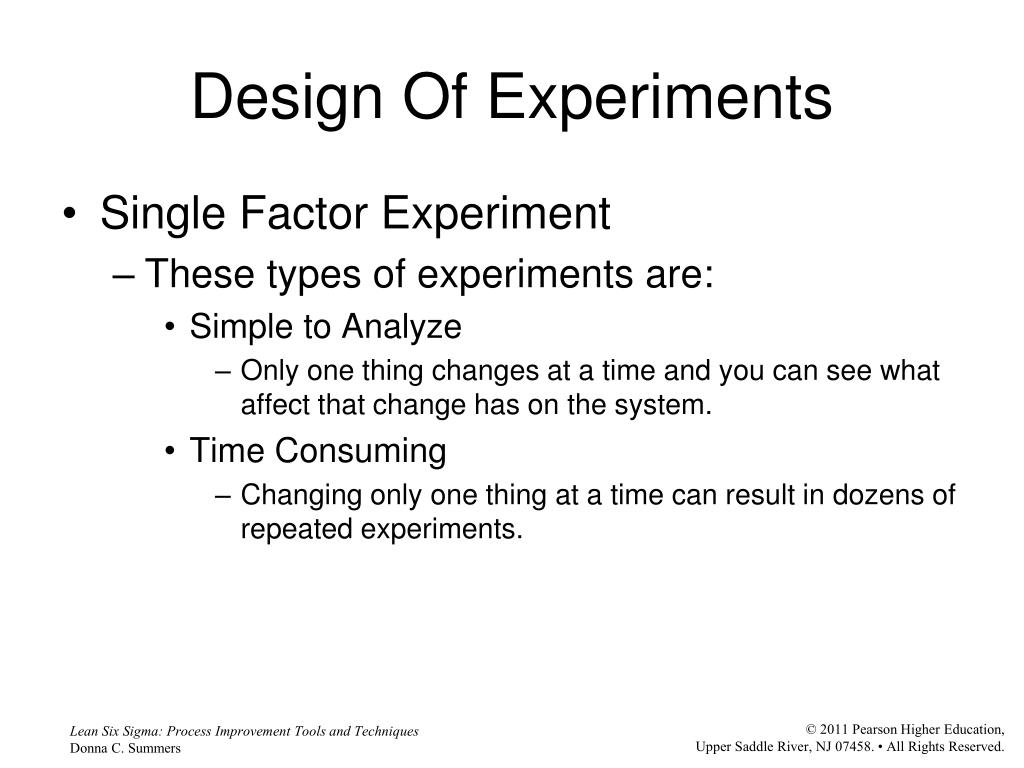
In this case, the inference made on the significance of the factor can be extended to the whole population but the factor effects are treated as contributions to variance. When we have missing data, it affects the average of the remaining treatments in a row, i.e., when complete data does not exist for each row - this affects the means. When we have complete data the block effect and the column effects both drop out of the analysis since they are orthogonal. With missing data or IBDs that are not orthogonal, even BIBD where orthogonality does not exist, the analysis requires us to use GLM which codes the data like we did previously. Here we have two pairs occurring together 2 times and the other four pairs occurring together 0 times.
How does blocking work in experimental design?
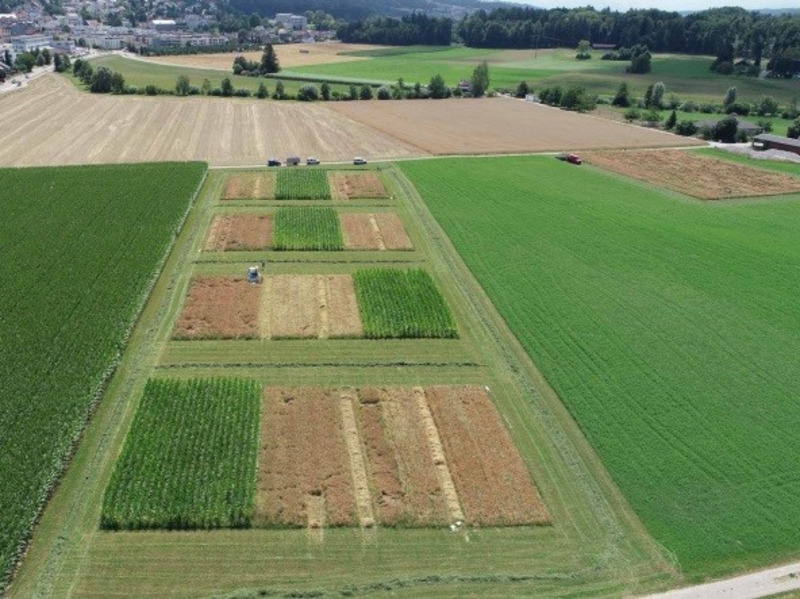
Note that the A effect and the B effect are orthogonal to the AB effect. This design gives you complete information on the A and the B main effects, but it totally confounds the AB interaction effect with the block effect. In this case treatment (1) and treatment ab will be in the first block, and treatment a and treatment b will be in the second block. Then randomly permute the order of the columns to yield the final square for the experimental layout. Where “i” is the index for replicates and “j” is the index for blocks within the replicates.
3 - Blocking in Replicated Designs
The general linear test is the most powerful test for this type of situation with unbalanced data. Here we have four blocks and within each of these blocks is a random assignment of the tips within each block. The nuisance factor they are concerned with is "furnace run" since it is known that each furnace run differs from the last and impacts many process parameters. The conf.design package in R is described by its author as a small library contains a series of simple tools for constructing and manipulating confounded and fractional factorial designs. The function conf.design can be used to construct symmetric confounded factorial designs. I could, of course, have someone else launch bears at the same time I do, so that I could get more data faster.
Example 4.1: Hardness Testing
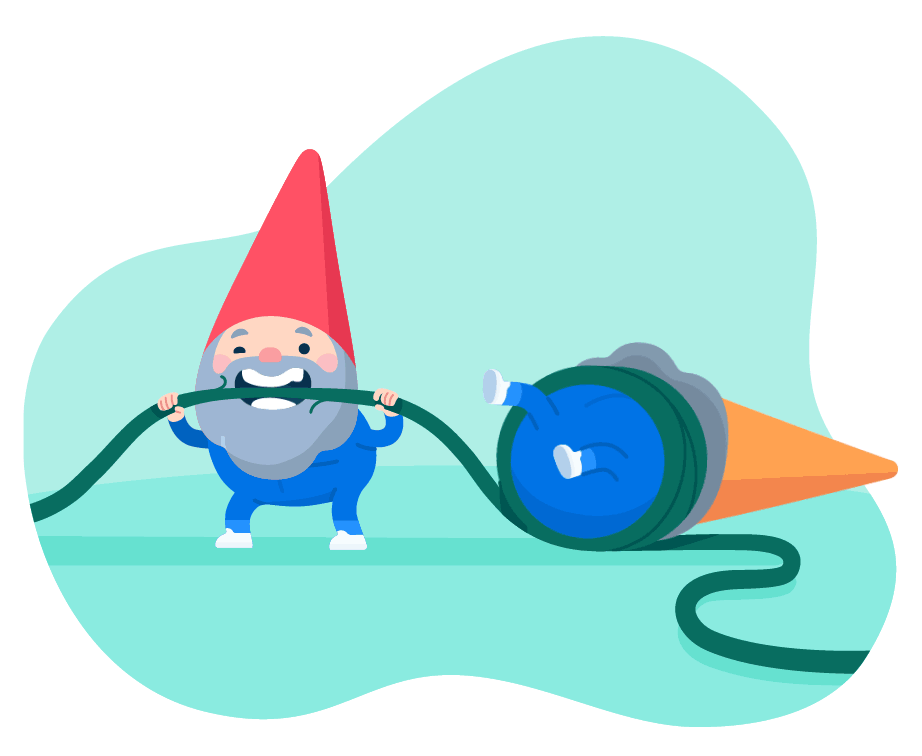
But I have other things to do besides launch gummi bears, so it's unlikely I can complete my experiment in one day. If I have to launch some bears on a day when it’s cold and rainy outside and some bears on a day when it’s sunny and mild, there might be an effect on how the gummi bears launch. BlockingWith small samples, it's possible to randomly divide the subjects and still get different groups. Randomly dividing the subjects into the 2 groups is the most likely to make the treatment and control groups as alike as possible because it eliminates human bias. Not only differences that the researcher has identified as relevant, but on all characteristics, including the hidden ones that the researcher might not realize are important.
In this case it would be near impossible to separate the impact that the type of cleats has on the run times from the impact that the type of field has.
Why is the randomized controlled double-blind experiment ideal?
Week one would be replication one, week two would be replication two and week three would be replication three. Variability between blocks can be large, since we will remove this source of variability, whereas variability within a block should be relatively small. In general, a block is a specific level of the nuisance factor.
Comparing the CRD to the RCBD
What we did here was use the one-way analysis of variance instead of the two-way to illustrate what might have occurred if we had not blocked, if we had ignored the variation due to the different specimens. Ok, with this scenario in mind, let's consider three cases that are relevant and each case requires a different model to analyze. The cases are determined by whether or not the blocking factors are the same or different across the replicated squares. The treatments are going to be the same but the question is whether the levels of the blocking factors remain the same. Therefore, one can test the block simply to confirm that the block factor is effective and explains variation that would otherwise be part of your experimental error. However, you generally cannot make any stronger conclusions from the test on a block factor, because you may not have randomly selected the blocks from any population, nor randomly assigned the levels.
Why have I been blocked?
Cryo-EM structures of ClC-2 chloride channel reveal the blocking mechanism of its specific inhibitor AK-42 - Nature.com
Cryo-EM structures of ClC-2 chloride channel reveal the blocking mechanism of its specific inhibitor AK-42.
Posted: Fri, 09 Jun 2023 07:00:00 GMT [source]
The reason for grouping experimental units is so that the observed differences between treatments will be largely due to “true” differences between treatments and not random occurrences or chance. If the number of times treatments occur together within a block is equal across the design for all pairs of treatments then we call this a balanced incomplete block design (BIBD). Here we have treatments 1, 2, up to t and the blocks 1, 2, up to b.
Procedure for Randomization
Here we have used nested terms for both of the block factors representing the fact that the levels of these factors are not the same in each of the replicates. We now illustrate the GLM analysis based on the missing data situation - one observation missing (Batch 4, pressure 2 data point removed). The least squares means as you can see (below) are slightly different, for pressure 8700.
For instance, if you had a plot of land the fertility of this land might change in both directions, North -- South and East -- West due to soil or moisture gradients. As we shall see, Latin squares can be used as much as the RCBD in industrial experimentation as well as other experiments. If the structure were a completely randomized experiment (CRD) that we discussed in lesson 3, we would assign the tips to a random piece of metal for each test.
The explanatory variable is the diet pill and the response variable is the amount of weight loss. Although the sex of the patient is not the main focus of the experiment—the effect of the drug is—it is possible that the sex of the individual will affect the amount of weight lost. The remaining four combinations are use in the second block of experiments.
The RCBD utilizes an additive model – one in which there is no interaction between treatments and blocks. The error term in a randomized complete block model reflects how the treatment effect varies from one block to another. Implementing blocking in experimental design involves a series of steps to effectively control for extraneous variables and enhance the precision of treatment effect estimates.
In our previous diet pills example, a blocking factor could be the sex of a patient. We could put individuals into one of two blocks (male or female). And within each of the two blocks, we can randomly assign the patients to either the diet pill (treatment) or placebo pill (control).
Comments
Post a Comment